Companies of all sizes are grappling with an ever-growing list of challenges: tough competitors, rapidly evolving customer preferences, and the constant pressure to do more with less. Artificial intelligence data analytics is transforming how businesses approach decision-making and strategy.
As AI advances, AI marketing tools are becoming indispensable for businesses aiming to enhance their competitive edge. From predicting market trends to automating complex data analysis, these tools offer unparalleled insights and efficiency.
Letβs explore how AI analytics revolutionizes business intelligence and how you can capitalize on these powerful tools to drive growth and innovation.
Table of Contents
What is AI analytics?
Why use AI for social media analytics
The business impact of AI analytics
AI analytics vs. Traditional analytics
The four pillars of AI analytics
Key elements of AI analytics
Big Data technologies
Top AI analytics tools
The future of AI analytics
What is AI analytics?
AI analytics applies artificial intelligence to quickly analyze large datasets, helping social media and marketing managers uncover valuable insights about their target audience persona with ease.
Unlike traditional methods, using AI for data analysis automates the process, allowing you to identify trends, understand audience behavior, and optimize campaigns in real time.
Whether you're tracking social media sentiment through social media analytics or predicting the success of a marketing strategy, AI-powered analytics tools give you the precision and speed needed to make smarter, data-driven decisions and stay ahead in a competitive landscape.
AI analytics offer businesses transformative insights by analyzing vast amounts of data quickly and accurately. This technology enables companies to make data-driven decisions, predict trends, and optimize operations.
Looking ahead, AI analytics is likely to become more sophisticated, integrating with other advanced technologies such as machine learning and natural language processing. This evolution will enhance predictive capabilities and offer more personalized customer experiences.
As AI continues to advance, businesses that leverage these tools effectively will gain a significant competitive edge, driving innovation and efficiency across various industries.β - Volkan Yolci, Business Developer at DemirΓΆren YatΔ±rΔ±m Holding
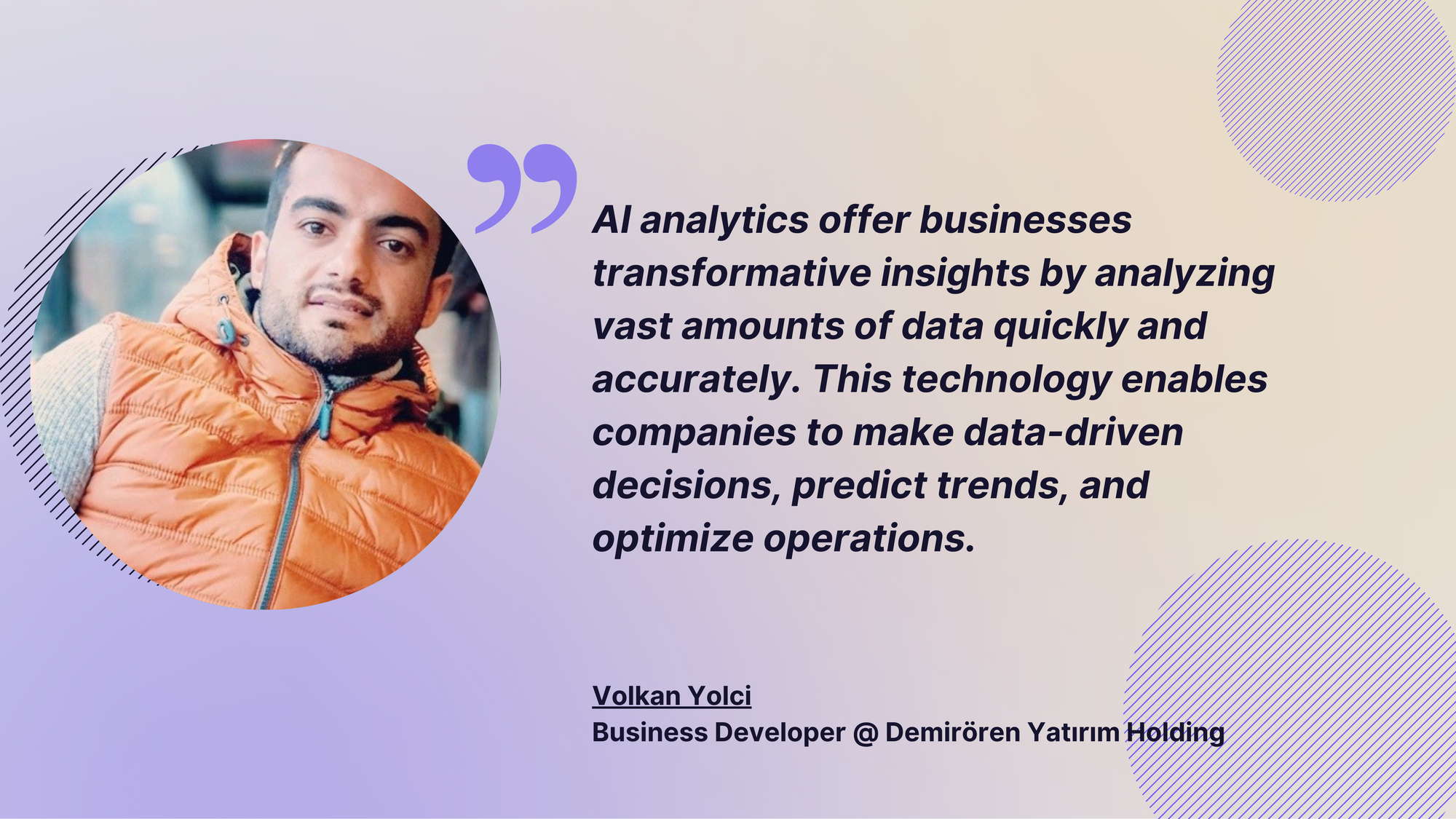
Why use AI for social media analytics?
AI analytics empowers social media and marketing managers to make faster, more informed decisions by automating data analysis, improving social media measurement, and uncovering insights that traditional methods might miss. Itβs the key to staying agile and ahead in todayβs competitive digital landscape.
Implementing AI analytics offers several advantages that can significantly enhance your social media and marketing strategies. Here are three key benefits.
Identify trends and patterns with AI analytics
Capturing and retaining consumer attention requires the ability to adapt quickly to changing trends and preferences.
AI analytics, including AI Instagram listening, empowers businesses to do just that by providing real-time insights into audience behavior.
AI uses machine learning and deep learning to predict future trends, allowing you to swiftly identify shifts in consumer preferences and adjust your content strategies and campaigns in real-time.
This agility ensures that your marketing efforts remain relevant and effective, aligning with your goals for social media, leading to sustained engagement and long-term success.
By staying in tune with your audience's evolving needs, you can outmaneuver competitors who rely on slower, more traditional methods of analysis.
Enhance coverage on decision-making through AI analytics
Traditional methods of gathering and analyzing audience insights often involved time-consuming processes, such as conducting surveys or focus groups, which could be expensive and slow to yield results.
AI analytics extracts meaningful insights by providing the ability to process vast amounts of data in real-time. This means that you can gather insights from multiple sources simultaneously, including social media insights, website interactions, and customer feedback, all at scale.
The speed and efficiency of AI analytics not only reduce costs but also enable more timely and impactful decision-making.
You can launch campaigns with confidence, knowing that they are backed by the latest data and insights, and you can quickly pivot if the data suggests a change in direction is needed.
Tap into hidden insights with predictive AI
One of the most powerful aspects of AI analytics is its ability to uncover AI insights that might not be immediately apparent through traditional methods.
AI-powered tools excel at identifying patterns and correlations within large, complex datasets, revealing opportunities and trends that may have otherwise gone unnoticed.
This capability allows marketers to explore beyond the usual questions and assumptions, often starting with a social media audit, leading to the discovery of new market segments, emerging consumer behaviors, and innovative strategies.
For example, AI might reveal that a particular product is unexpectedly popular among a demographic that wasnβt initially targeted, or provide insights through social media competitor analysis that can influence strategy, or that a specific type of content consistently drives higher engagement at certain times.
By tapping into these hidden insights, businesses can develop more creative and effective marketing strategies that set their brand apart in a crowded marketplace.
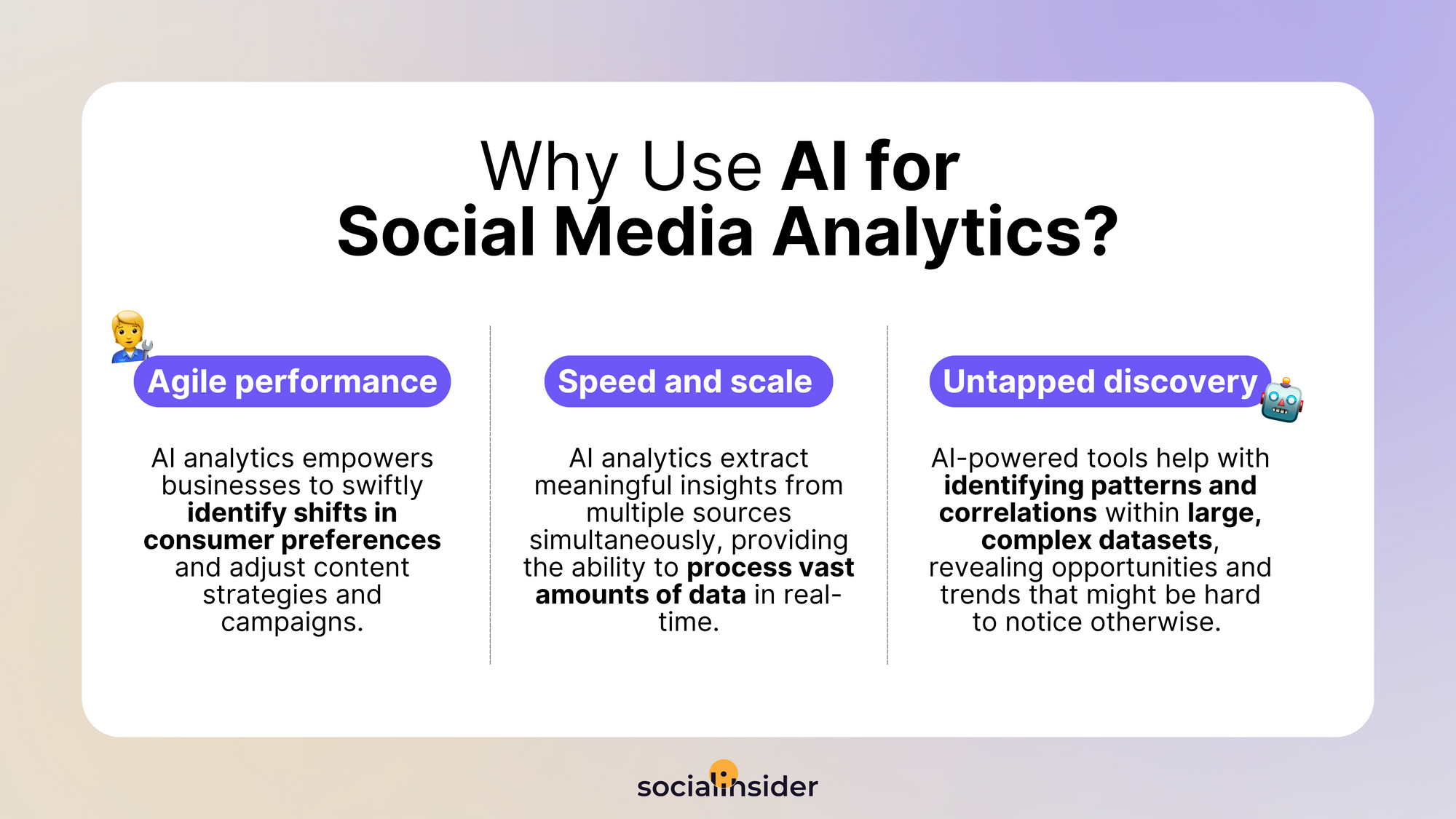
The business impact of AI analytics
AI analytics is transforming business operations by providing deeper competitive insights and improving personalization. AI is transforming how businesses are operating by helping them automate tasks. Hereβs how businesses can leverage these tools.
Get customer insights and personalization
With the rising demand for AI analytics, businesses can better understand their customers by analyzing data on behavior and preferences, which can significantly enhance brand awareness efforts. This enables personalized marketing campaigns, boosting engagement and loyalty.
Analyze sentiment on social media across all regions and platforms
Business applications of AI analytics include tools allows businesses to track public sentiment in real-time, playing a crucial role in brand reputation management. By monitoring social media and online content, companies can quickly identify trends and adjust strategies to maintain a positive brand image.
Categorize audience messages
AI can score and categorize audience messages based on sentiment and content. This helps prioritize responses, ensuring critical customer interactions are handled efficiently, and enhancing customer satisfaction.
Identify anomalies through data
AI social listening tools are crucial for detecting and addressing false information quickly. By scanning online content for inaccuracies, businesses can protect their brand from potential reputational damage.
Improve demand forecasting
AI analytics improves demand forecasting by analyzing historical data and market trends. This allows businesses to optimize inventory, plan marketing efforts, and allocate resources more effectively.
Monitor business metrics
AI analytics gives a more comprehensive report on continuous performance by providing real-time insights into key performance indicators (KPIs). This continuous monitoring, enhanced by social media insights, helps businesses track performance, identify areas for improvement, and make informed decisions quickly.
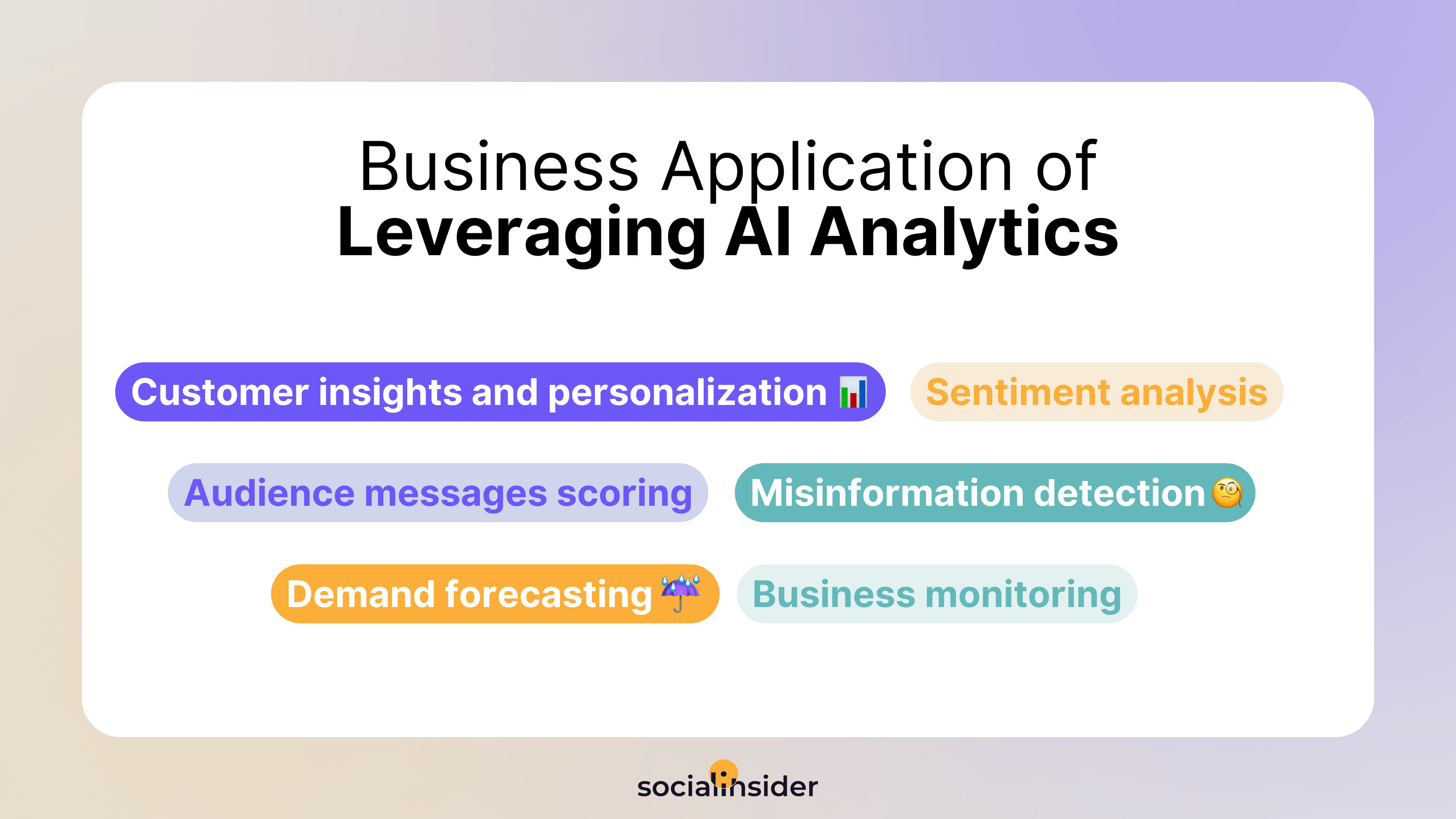
AI analytics vs Traditional analytics
As businesses increasingly rely on data to inform their decisions, the debate between AI analytics and traditional analytics becomes more prominent.
While both approaches offer valuable insights, AI analytics provides distinct advantages that set it apart from traditional methods, particularly in speed, efficiency, and the ability to handle complex data.
One common question is, "can AI analyze data more effectively than traditional methods?"
The answer is a resounding yes.
AI analytics automates data processing, significantly speeding up analysis and allowing businesses to quickly gain insights and react to market changes in real-time.
Pros and Cons of AI analytics vs. Traditional analytics
Speed and efficiency
Traditional analytics often involves manual processes, such as data cleaning, model building, and interpretation, which can be time-consuming, especially when dealing with large datasets. ML-based automation can significantly reduce this burden.
For example, analyzing a dataset from multiple marketing campaigns might take weeks using traditional methods, delaying critical decision-making processes. In contrast, Artificial Intelligence analytics capabilities automate these steps, significantly speeding up the analysis.
With AI, businesses can process large datasets in a fraction of the time, enabling them to gain insights rapidly and react to market changes in real-time. This speed is a significant competitive advantage, especially in fast-paced industries like retail and digital marketing.
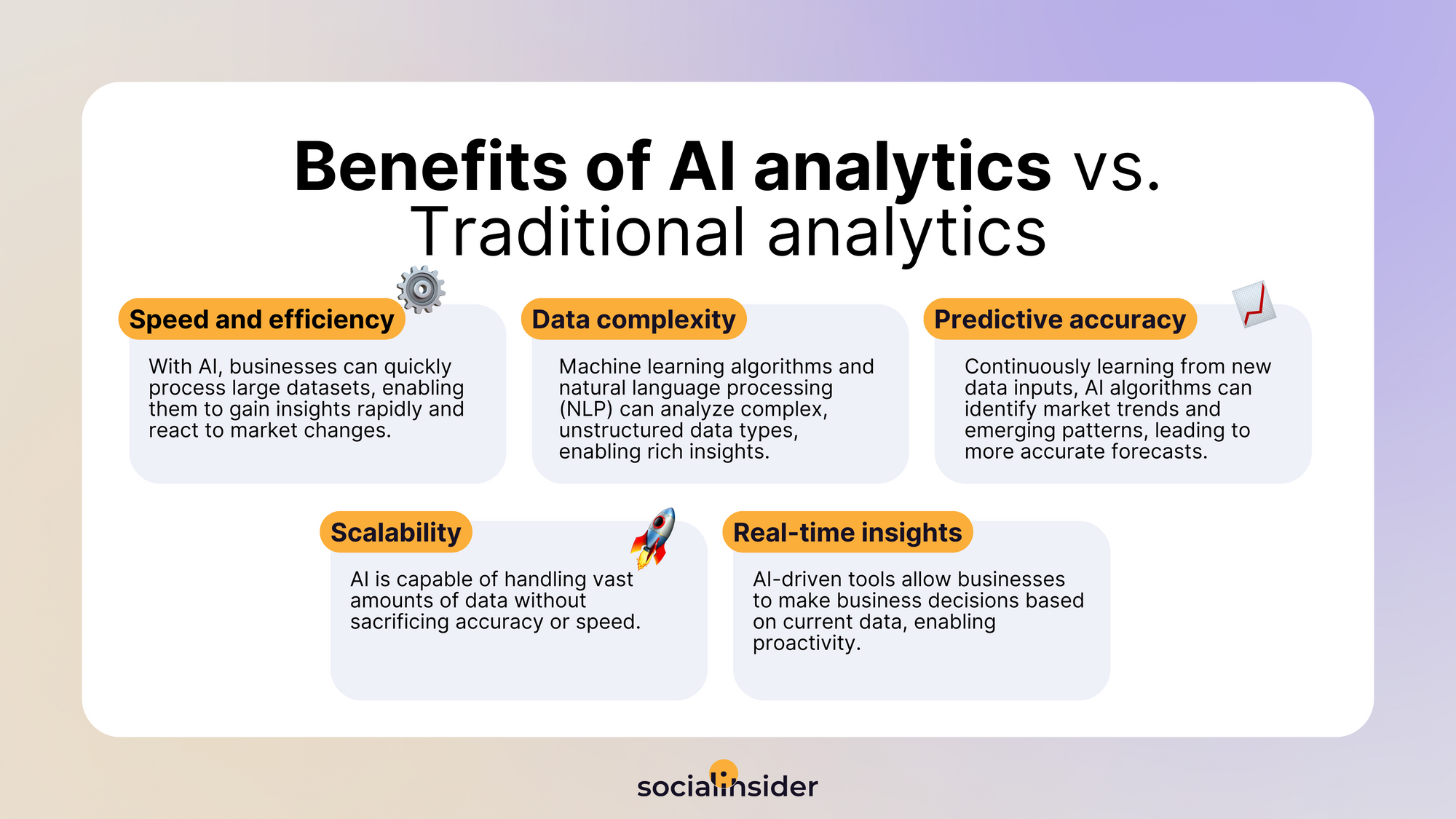
Data complexity
Traditional analytics can struggle with analyzing unstructured data, such as social media posts, customer reviews, or multimedia content.
These data types are often rich in insights but challenging to quantify and analyze using traditional tools.
For example, a company trying to gauge public sentiment from social media might find it difficult to accurately interpret the context and nuances of language using conventional analytics methods.
AI analysis excels in this area by employing machine learning algorithms and natural language processing (NLP) to analyze complex, unstructured data types.
AI automates repetitive and time-consuming tasks involved in data analysis, allowing businesses to gain a more comprehensive understanding of consumer behavior and market trends, leading to more informed strategic decisions.
AI has been part of GA4 for years, but that βAnalytics Intelligenceβ feature isnβt very useful. I donβt know anyone who uses it. But marketers who have tried exporting data from GA4 (or any tool with data) and uploading it to ChatGPT (or any AI) are finding interesting insights.
For example, if you add βdateβ as a secondary dimension to a GA4 report showing key events, the AI can make a chart showing your conversions by day of week. GA4 alone canβt do that. Once you realize whatβs possible when you upload marketing data to AI, youβll get excited anytime you see an βExportβ button! - Andy Crestodina, Co-Founder and CMO at Orbit Media
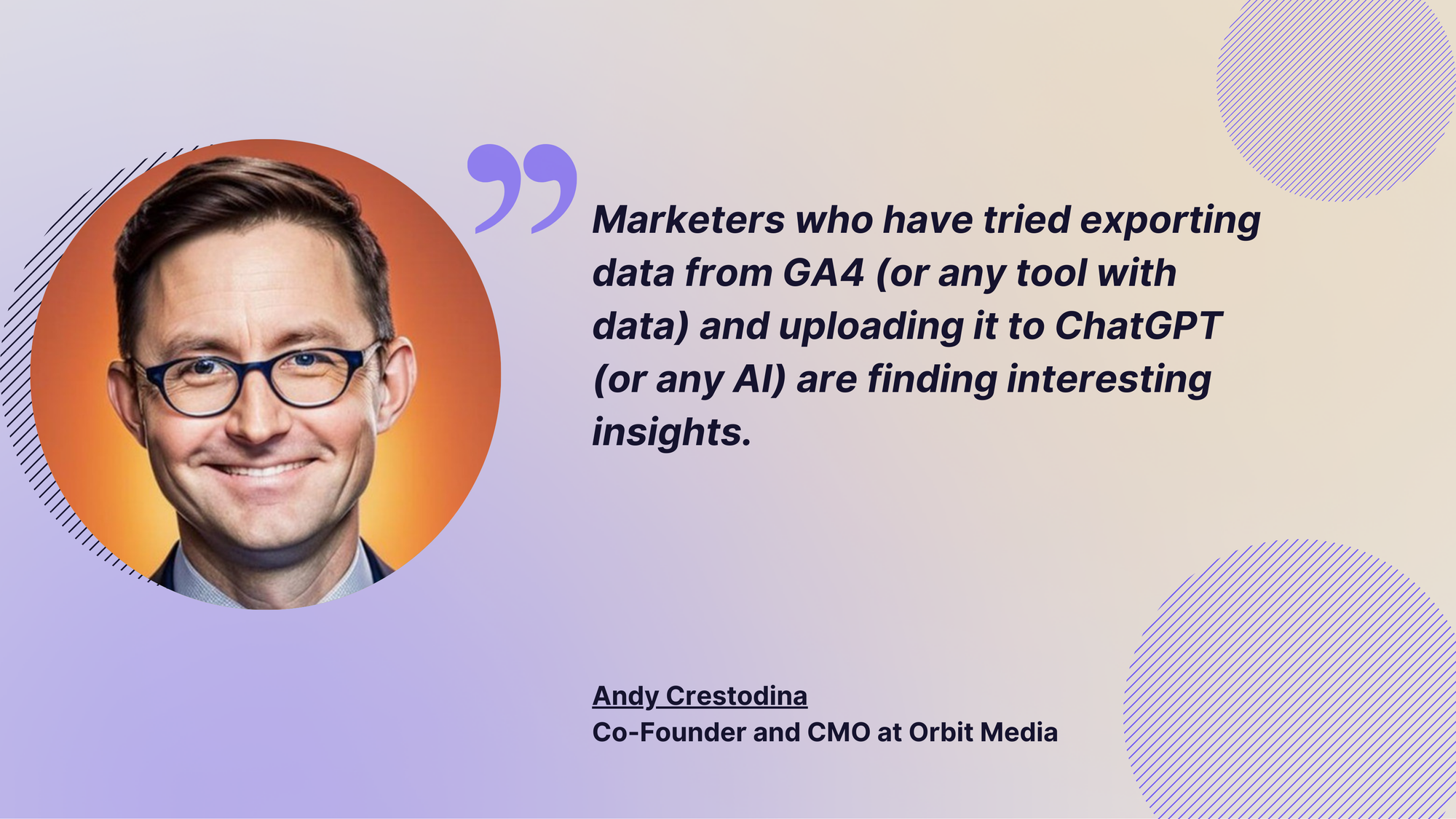
Predictive accuracy
While traditional analytics relies on historical data to make predictions, it often uses static models that don't account for new data or changing conditions. This can lead to outdated or less accurate forecasts.
In contrast, AI analytics enhances the predictive process by continuously learning from new data inputs.
AI algorithms can identify market trends, customer segments, and emerging patterns, leading to more accurate forecasts and better-informed business decisions.
For instance, an AI model could predict customer churn more accurately by continuously analyzing recent interactions, purchasing behaviors, and external factors like economic shifts.
Scalability
As businesses grow and data volumes increase, traditional analytics can become cumbersome, often requiring significant human resources to manage and analyze the data. This can lead to inefficiencies and bottlenecks, particularly in large organizations where data is generated at high volumes across multiple departments.
AI can enhance analytics and business intelligence by being inherently scalable and capable of handling vast amounts of data without sacrificing accuracy or speed. This scalability is crucial for businesses looking to expand and adapt in a data-driven world, allowing them to process and use AI to analyze data from a growing number of sources efficiently.
Real-time insights
Traditional analytics typically provides insights based on past data, which can delay decision-making.
For example, a traditional analysis might show that a marketing campaign performed well last quarter, but by the time this insight is gathered, the market conditions may have changed.
AI can analyze large volumes of historical and real-time data, offering real-time insights that allow businesses to make proactive adjustments to their strategies as new data becomes available. This capability is particularly valuable in dynamic markets where conditions can change rapidly, enabling businesses to stay agile and responsive.
Examples where traditional analytics fall short
Traditional analytics struggle to analyze the vast amount on unstructured data
One clear example of where traditional analytics falls short is in sentiment analysis on social media platforms. Traditional analytics might struggle to analyze the vast amount of unstructured data generated by user comments, reviews, and posts.
AI-enabled automation to optimize and see the ROI of your social and digital data can address these challenges. Traditional methods might miss the context of analyzing trends and anomalies, failing to provide report summaries.
With its advanced natural language processing capabilities, AI analytics can predict social media content performance, compare it with competitors, and provide data-driven strategies.
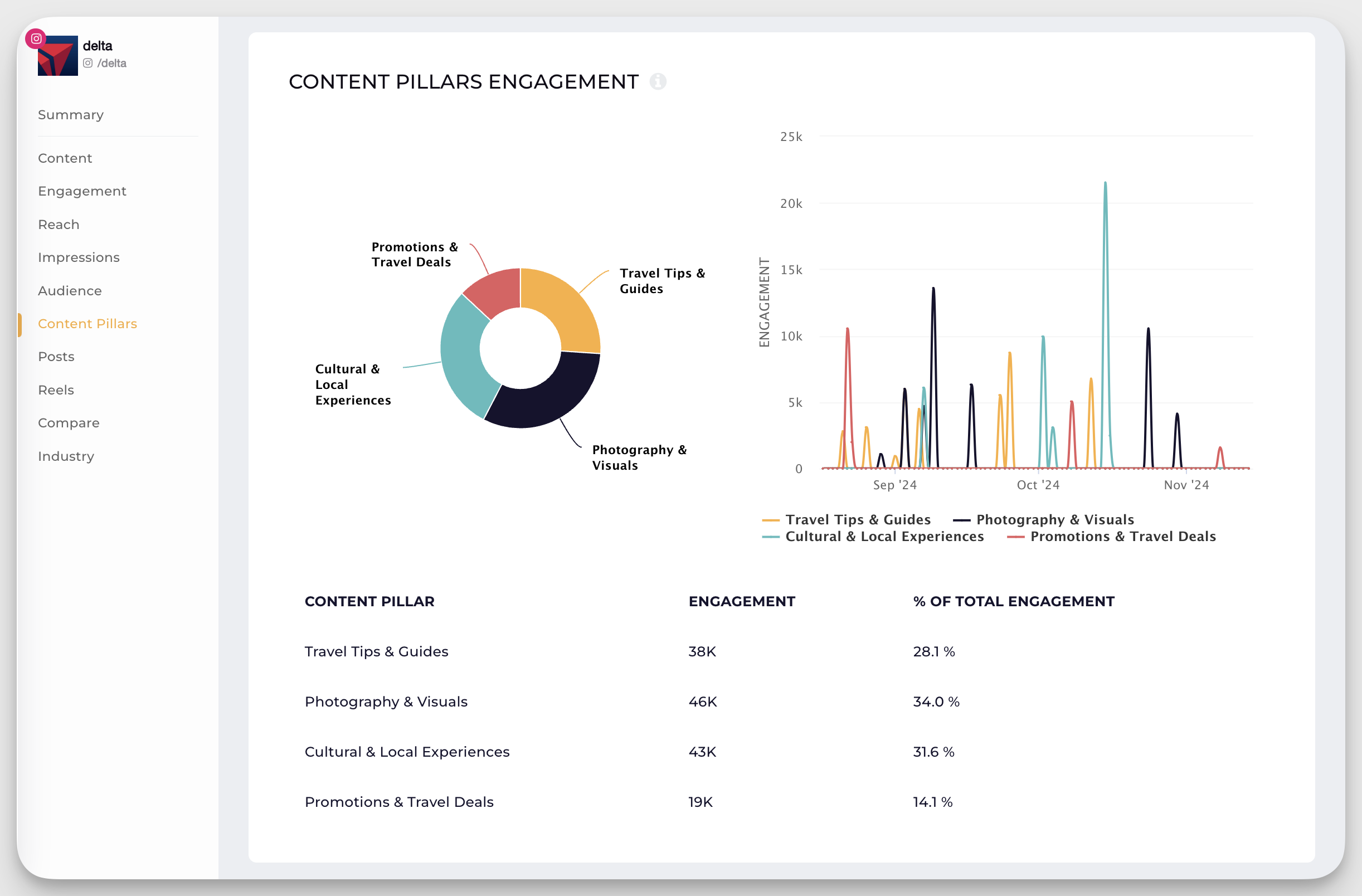
Traditional analytics use historical data to predict the future
Another example is in predictive maintenance for manufacturing. Traditional analytics might use historical data to predict when a machine will fail, but this approach often relies on static models that donβt adapt well to new data or changing conditions.
AI analytics, however, continuously learns from real-time data streams, allowing it to predict failures more accurately and in real-time, thereby reducing downtime and maintenance costs.
Transitioning from traditional to AI
Transitioning from traditional analytics to AI analytics is not without its challenges, but the benefits far outweigh the difficulties. One of the primary challenges is the integration of AI tools with existing systems.
Many businesses have invested heavily in traditional analytics platforms, and transitioning to AI requires not only technological integration but also a shift in mindset and culture.
When evaluating data analytics platforms, itβs essential to consider how well they support this transition, including their ability to integrate with existing systems and their potential to deliver advanced insights and efficiencies.
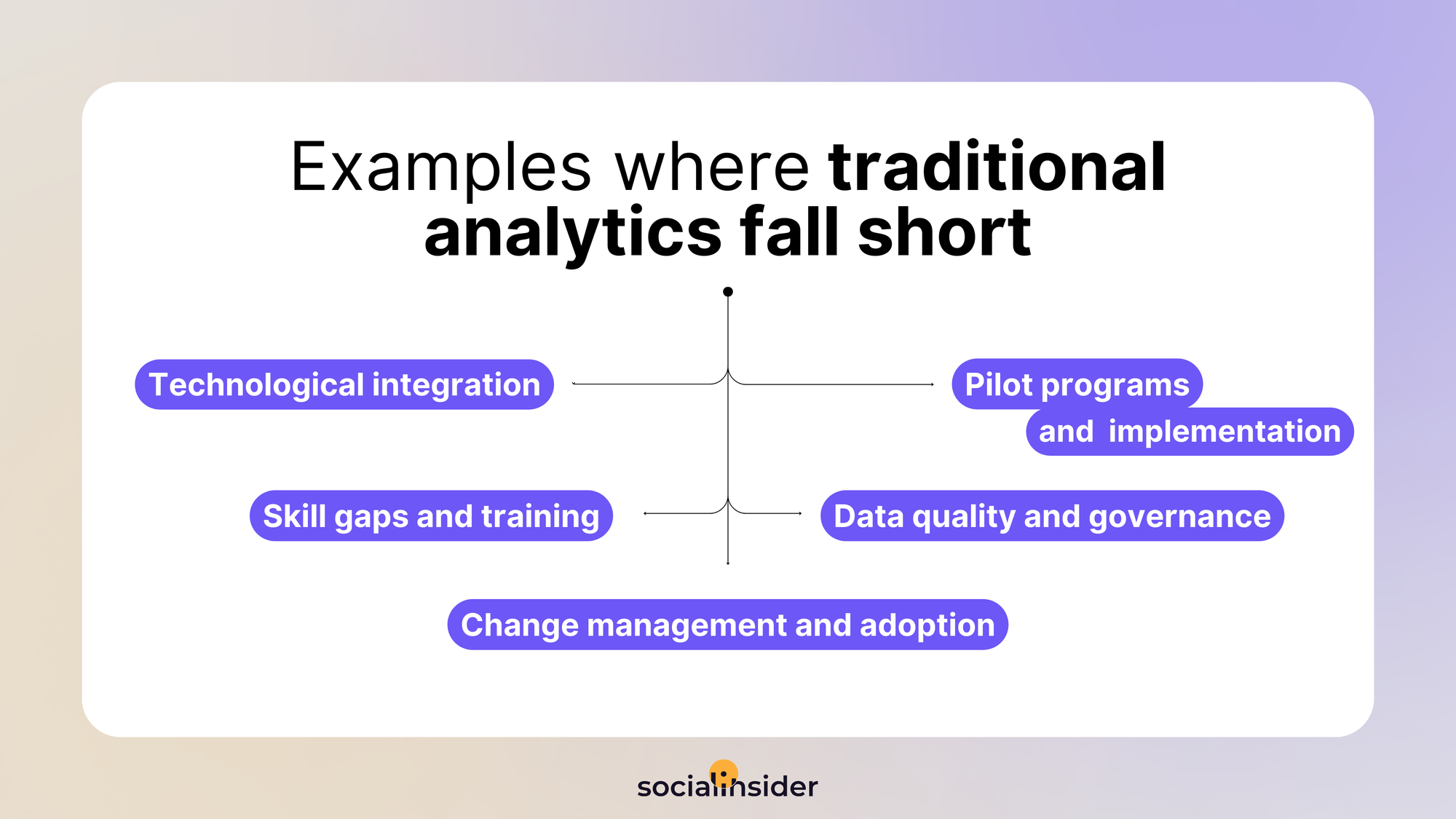
Integrate new technologies to work with AI
Integrating AI analysis tools with existing data infrastructure can be complex. Businesses need to ensure that their current data warehouses, databases, and reporting tools are compatible with AI technologies.
This might involve upgrading existing systems or adopting new platforms that support AI-driven analytics. Additionally, businesses need to invest in the right data management tools that can handle the volume, variety, and velocity of data required for AI analytics.
Learning new skills to work with AI analytics
Another challenge is the skill gap within the workforce. Traditional analytics relies heavily on human intervention, with data scientists and analysts playing a central role in interpreting data. AI analytics, however, requires a different set of skills, including knowledge of machine learning, data science, and AI technologies.
The power of AI lies in its ability to automate complex analyses and generate insights with minimal human intervention, which underscores the need for training programs that bridge these skill gaps and prepare teams to harness AI effectively
Businesses must invest in training their workforce to bridge this gap or hire new talent with the necessary skills. This might also involve restructuring teams to include AI specialists who can lead the transition and ensure the effective deployment of AI tools.
Change management and new behaviors to integrate AI
The transition to AI analytics also requires effective change management.
Employees who are accustomed to traditional analytics methods might be resistant to adopting new AI tools, especially if they feel their jobs are at risk.
To overcome this, businesses should focus on the benefits of AI, such as reduced workload, enhanced decision-making capabilities, and the ability to focus on more strategic AI tasks.
Encouraging a culture of innovation and continuous learning can help ease this transition, making employees more receptive to AI technologies.
Get trust in AI analytics data
Data quality and governance are critical for the success of Artificial Intelligence analytics. Poor data quality can lead to inaccurate insights, undermining the effectiveness of AI tools.
Businesses need to establish robust data governance frameworks that ensure data accuracy, consistency, and security. This includes implementing data cleaning processes, setting up data management policies, and ensuring compliance with regulations like GDPR.
Run pilot programs and gradual implementation
To mitigate these challenges, businesses can start by implementing pilot programs that allow them to test AI analytics tools, including those utilizing augmented analytics, on a smaller scale before rolling them out across the organization.
This approach helps identify potential issues early and allows for adjustments before full-scale implementation. Gradual implementation also gives employees time to adapt to the new tools and processes, making the transition smoother.
The four pillars of AI analytics
AI analytics is built on four foundational technologies that enable businesses to extract valuable insights from vast and complex datasets. These pillars - machine learning, natural language processing, neural networks, and deep learning - work together to provide a comprehensive and powerful approach to data analysis.
The integration of AI in business analytics allows companies to leverage these technologies to not only analyze historical data but also to predict trends, optimize processes, and make more informed decisions.
Machine Learning
Machine learning (ML) is at the core of AI analytics, enabling models to learn from historical data and make predictions about future outcomes.
For businesses looking to understand how to use AI for data analysis, the process begins with selecting the appropriate AI model based on the specific problem and characteristics of the data.
Machine learning algorithms and similar AI tools have been in the toolkit of data analysts for quite some timeβthey required more intimate knowledge on how they work and expertise to be able to use them. If you werenβt a data scientist you had no chance to use those tools.
The current state of AI has made it possible to dive into the data easier, with minimum frictionβyou now get a tool thatβs going to make it fun to get more data into your decisions.
As it evolves, analytics will shift from providing the numbers to providing advice and predictions to help you achieve your goalsβyour very own strategy assistant.β Β - Andrei Serbanoiu, Co-Founder at Socialinsider
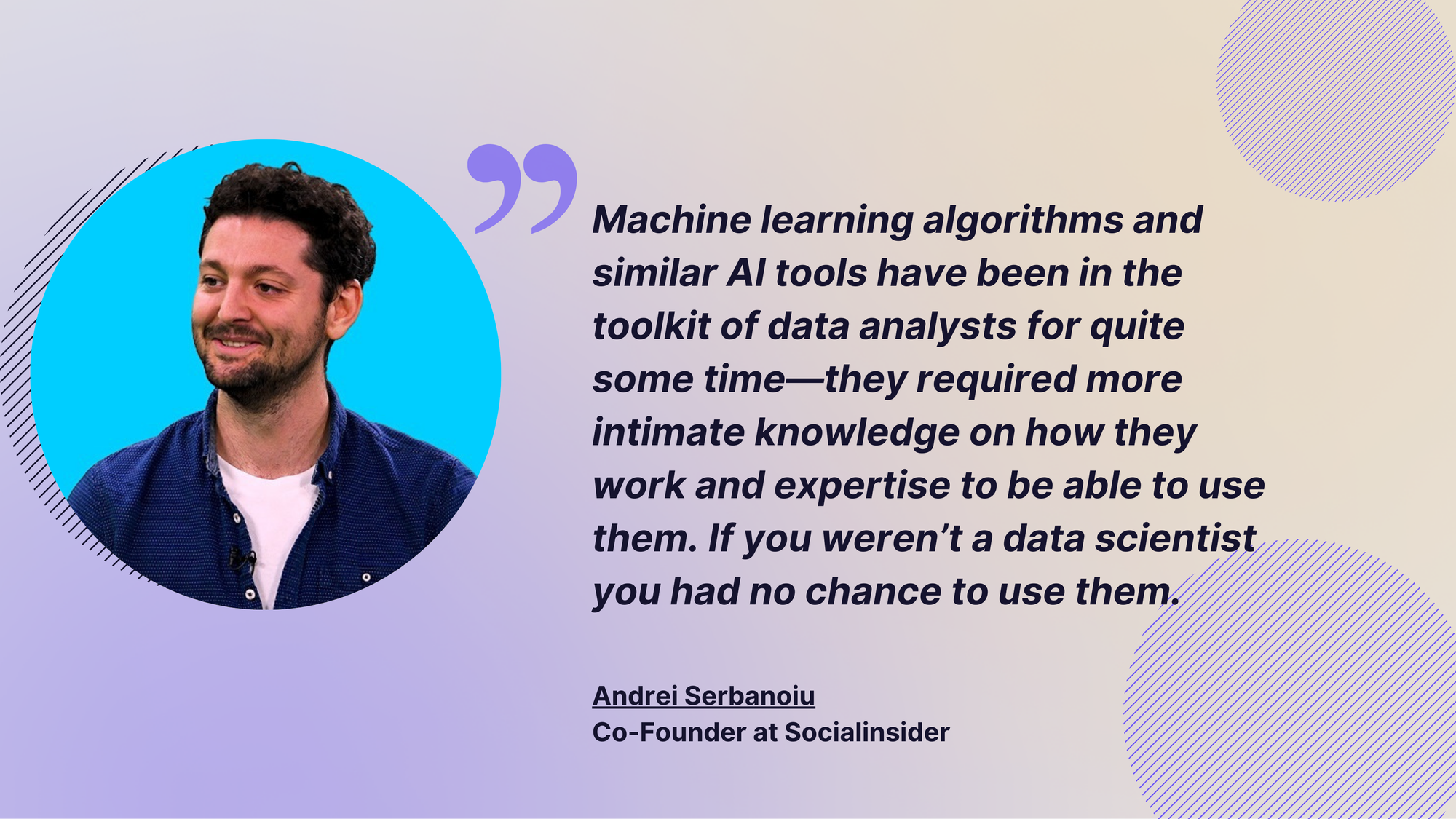
Natural Language Processing (NLP)
NLP is essential for analyzing and interpreting human language. It supports data analytics by allowing AI analytics tools to process and understand text-based data, such as social media posts, customer reviews, and emails.
By leveraging NLP, businesses can gain insights into customer sentiment, identify emerging topics, and respond more effectively to audience needs.
Neural Networks
Inspired by the human brain, neural networks are a series of algorithms that recognize relationships in data. These networks are particularly useful in identifying complex patterns and correlations that traditional analytics might miss.
In AI analytics, neural networks are used to enhance predictive accuracy and uncover deeper insights from large datasets.
Deep Learning
A subset of machine learning, deep learning involves the use of advanced neural networks with multiple layers (hence the term "deep").
Deep learning is capable of processing vast amounts of unstructured data, such as images, videos, and text, to generate highly detailed and nuanced insights. This makes it a powerful tool for businesses looking to analyze complex data and make data-driven decisions.
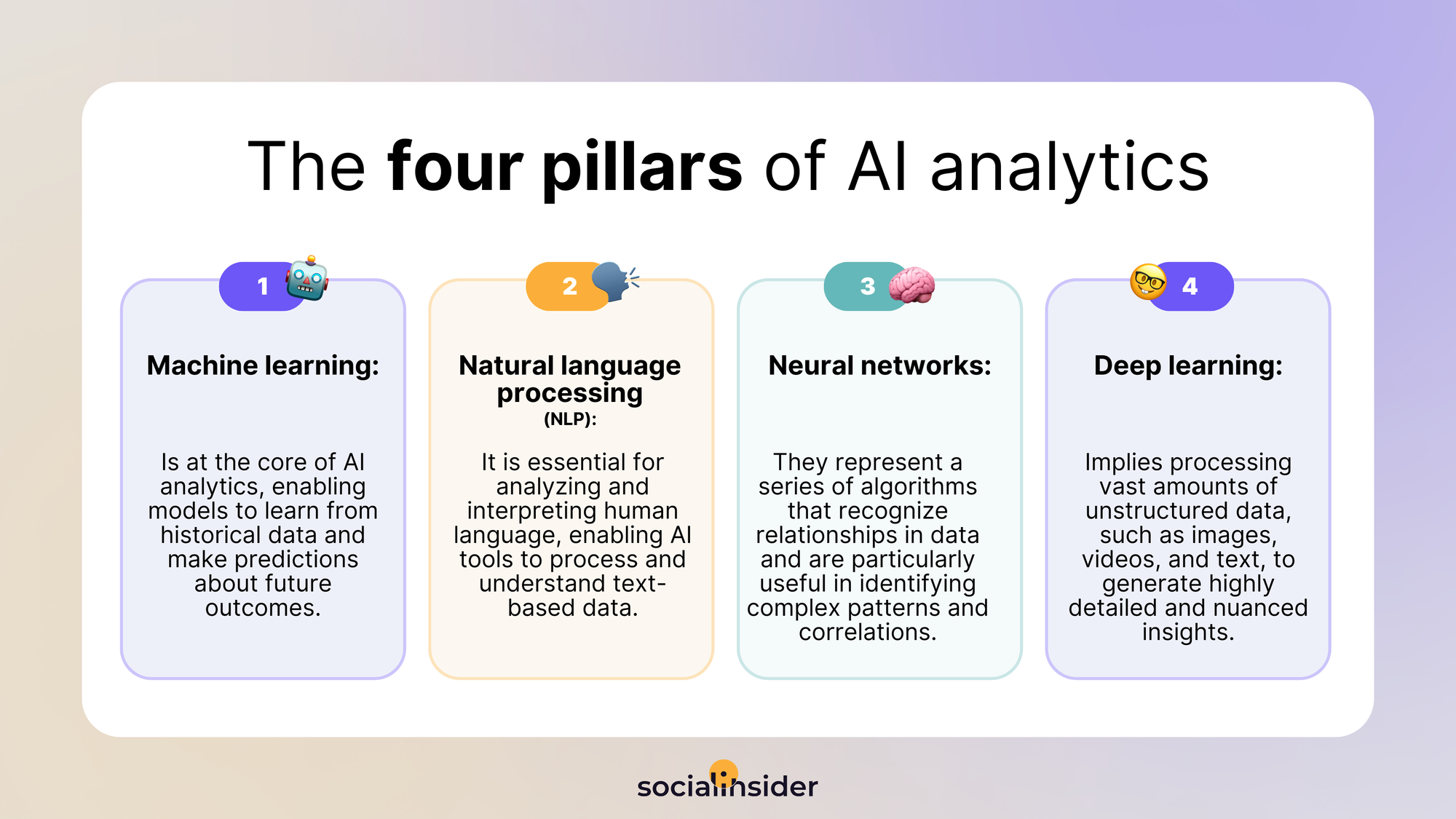
Key elements of AI analytics
AI analytics has emerged as a game-changer in the business world, offering unparalleled capabilities for processing and interpreting vast amounts of data. However, the effectiveness of AI analytics relies heavily on several key elements that work in unison to deliver accurate, actionable insights.
Understanding how to use AI in data analytics is crucial for businesses looking to harness the full potential of AI in their data analysis efforts.
Data collection and integration
The effectiveness of AI analytics starts with comprehensive data collection and integration.
AI enhances data collection by automating the process of gathering data from various sources, including databases, social media, sensors, and APIs, to provide a holistic view of business operations and customer behavior. However, merely collecting data isnβt sufficient; integrating this data into a unified dataset is crucial.
Integration ensures that all data points are consistent and complete, allowing AI models to analyze the information accurately. This unified approach enables businesses to make more informed decisions and derive better predictions from their AI tools.
Data preparation
Once data is collected and integrated, the next critical step is data preparation. This process is essential for ensuring that the data is clean, accurate, and ready for analysis.
For businesses looking to understand how to use AI to analyze data, data preparation is a crucial starting point. It involves several key processes:
- Data cleaning: This process involves identifying and correcting errors or inconsistencies in the data. Common issues include missing values, duplicate records, and outliers that can distort the analysis. By cleaning the data, businesses ensure that the dataset is reliable and free from errors that could lead to incorrect conclusions.
- Data transformation: After cleaning, the data must be transformed into a format that is suitable for analysis. This involves processes like normalization (scaling the data to a standard range), encoding (converting categorical data into numerical format), and aggregation (summarizing data points). Data transformation is crucial because it makes the data compatible with AI models, enabling them to process and analyze it effectively.
- Feature engineering: Feature engineering is the process of creating new variables (features) from the raw data that can help improve the performance of AI models. For example, in a sales dataset, new features like 'average purchase value' or 'purchase frequency' can be derived from existing data.
These features provide additional context and can help the AI models identify patterns and generate more accurate insights. An AI analytics example of this is using these engineered features to better predict customer lifetime value or to optimize sales strategies based on refined data insights.
Efficient data preparation maximizes the accuracy and effectiveness of AI analytics, ensuring that the insights generated are based on high-quality data. This step is particularly important because even the most advanced AI models will produce poor results if the underlying data is flawed.
Machine Learning and AI models
Machine learning (ML) is at the core of AI analytics, enabling models to learn from historical data and make predictions about future outcomes. The process begins with selecting the appropriate AI model based on the specific problem and characteristics of the data.
The model is then trained using historical data to recognize patterns and make predictions.
After training, the modelβs performance is validated on a separate dataset to ensure it can be generalized to new data.
Finally, hyperparameters are fine-tuned to optimize the modelβs accuracy and efficiency. By carefully managing these steps, businesses can leverage AI and ML to enhance their data analysis capabilities and support more informed decision-making.
Advanced AI analytics techniques
In todayβs data-driven world, the integration of data analytics and AI has revolutionized how businesses extract insights and make decisions.
AI analytics employs several advanced techniques to delve deeper into data, helping businesses make more informed choices.
Predictive analytics: This technique uses AI models to forecast future outcomes based on historical data, allowing businesses to anticipate trends and prepare accordingly.
Prescriptive analytics: Building on predictive insights, prescriptive analytics provides actionable recommendations on the best steps to take, optimizing decision-making processes.
Descriptive analytics: By summarizing historical data, descriptive analytics helps businesses understand past trends and patterns, often visualized through a social media dashboard, offering a clear view of what has occurred.
Diagnostic analytics: This approach digs into the data to determine the root causes of past events, enabling businesses to learn from their history and avoid future issues.
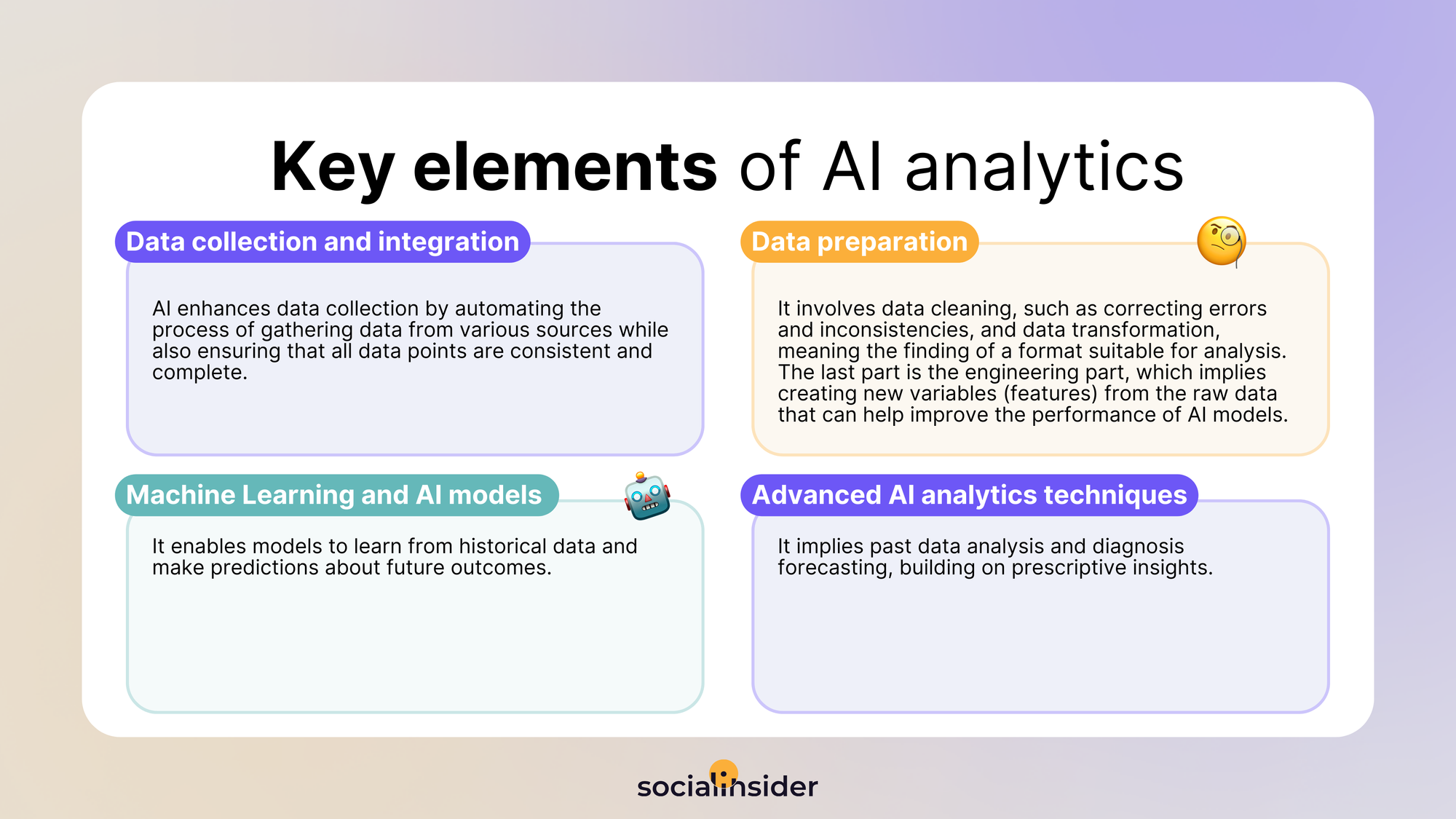
Big Data technologies
Handling large datasets is a critical aspect of AI analytics, and big data technologies provide the necessary infrastructure.
AI analytics relies on robust storage solutions like Hadoop and Spark to manage and store massive volumes of data. These databases and data warehouses ensure that all relevant data is readily accessible for analysis.
To efficiently process and analyze big data, AI-powered analytics tools use distributed computing frameworks. These frameworks, such as those built on Hadoop and Spark, enable quick and scalable analysis, allowing businesses to extract valuable insights from vast datasets.
By integrating these big data technologies, companies can fully leverage AI analytics to handle complex data challenges and drive informed decision-making.
Visualization and interpretation
Effective visualization and interpretation are key to making AI analytics insights actionable.
AI in analytics plays a critical role in transforming complex data into visual formats that are easy to understand and interpret.
By using AI-driven tools, businesses can create visual representations of data and model results, making it easier for decision-makers to grasp key insights quickly.
Interactive dashboards and reports are developed to communicate findings to stakeholders in a user-friendly format. These tools allow decision-makers to quickly grasp key insights and make informed choices based on the data.
Deployment and monitoring
Efficient AI analytics requires seamless deployment and ongoing monitoring of models to maintain accuracy and relevance.
The integration of AI and data analytics into production systems enables businesses to make real-time predictions and informed decisions based on up-to-date insights.
Continuous monitoring of model performance is crucial to detect any drifts or changes in data patterns.
AI models can analyze historical data to detect anomalies, and regular updates and adjustments are made to keep the models accurate and effective, ensuring they continue to deliver reliable insights.
Ethics and compliance
Maintaining ethics and compliance in AI analytics is crucial for building trust and ensuring legal compliance.
AI models must be designed to be free from bias, ensuring fair and equitable results across different demographic groups. This is essential for avoiding discrimination and maintaining the integrity of AI-driven decisions.
Additionally, protecting sensitive data is a top priority, especially in an era of stringent privacy regulations like GDPR.
Businesses must ensure that their AI models comply with these regulations, safeguarding personal information and upholding customer trust. By focusing on ethics and compliance, AI analytics not only delivers valuable insights but also maintains the highest standards of data security and ethical responsibility.
Scalability and performance
Scalability and performance are essential for the long-term success of AI analytics.
As businesses grow, their AI analytics systems must be able to handle increasing amounts of data and a growing number of users without compromising on efficiency or accuracy.
Scalable AI-powered analytics solutions allow businesses to expand their data operations seamlessly, ensuring that insights remain reliable and accessible even as data volumes increase.
Continuous improvements in data processing speed and model inference are also necessary to keep AI models running smoothly and delivering timely, accurate AI-generated insights.
By focusing on scalability and performance, businesses can ensure that their AI analytics solutions will continue to meet their needs as they evolve.
Human-AI collaboration
The future of AI in analytics lies in the collaboration between human expertise and AI-enabled data for AI marketing.
By combining AIβs ability to handle data-intensive tasks with human intuition and strategic thinking, businesses can enhance their decision-making processes.
AI can automate the analysis of large datasets and provide recommendations, while humans can interpret these insights and make strategic decisions based on their experience and understanding of the business context.
AI analysis helps businesses automate statistical calculations to monitor key performance indicator (KPI) metrics across platforms. Developing AI models that provide clear and interpretable insights is essential for building trust and ensuring adoption across the organization.
Explainable AI makes it easier for users to understand how decisions are made, fostering confidence in the use of AI-powered analytics and encouraging a collaborative approach to data-driven decision-making.
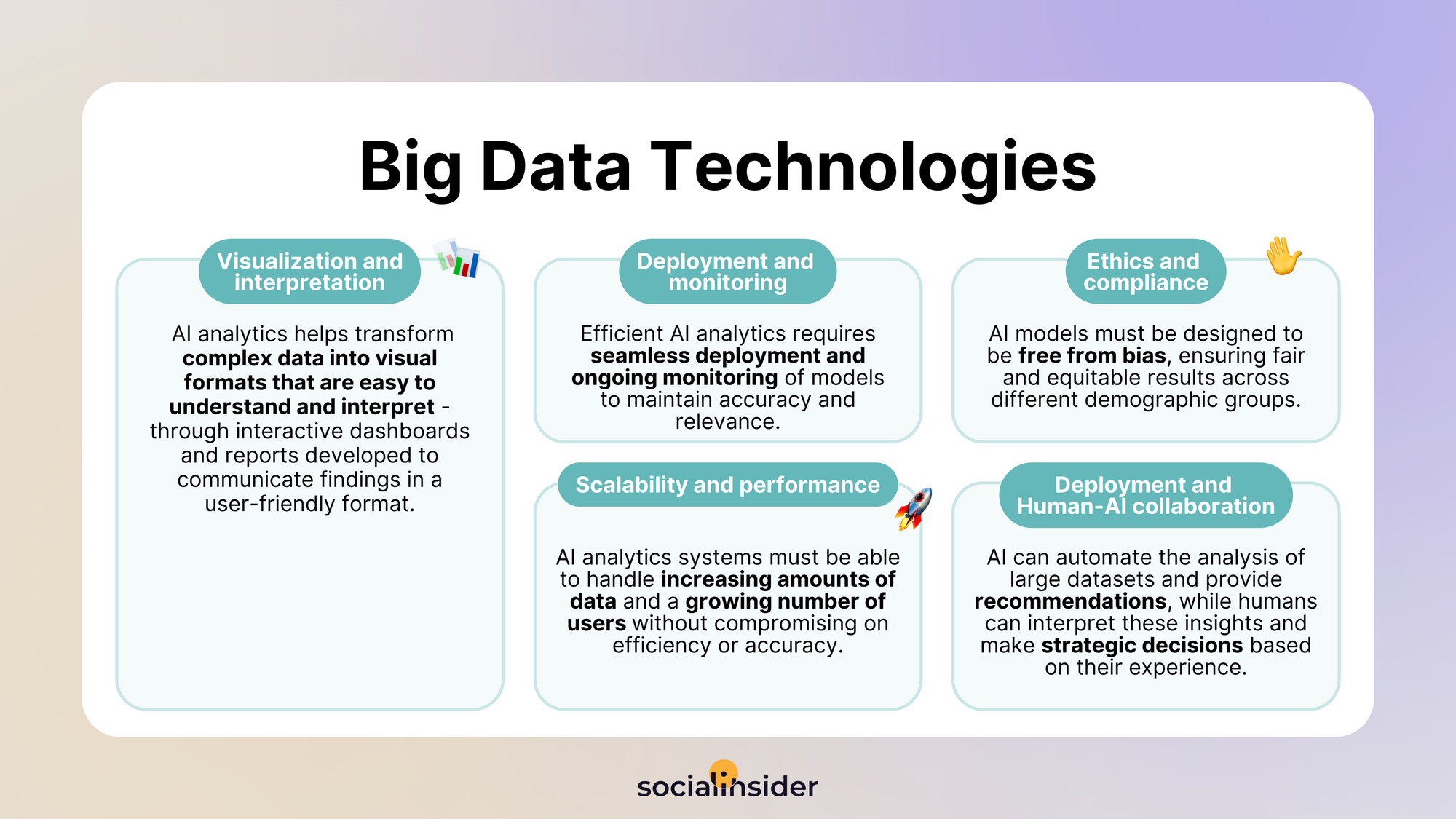
Top AI analytics tools
Socialinsider (for content analysis)
Socialinsider is a valuable tool for businesses aiming to gain a competitive edge through comprehensive competitive intelligence powered by AI and data analysis.
Leveraging AI analytics provides in-depth insights into competitors' social media strategies, including engagement metrics, posting frequencies, and content performance.
This allows you to benchmark your brand against competitors, identify gaps in your strategy, and capitalize on opportunities that might otherwise go unnoticed.
By using AI for content analysis, business can adjust their content strategies and get clear insights into what's working and what's not.
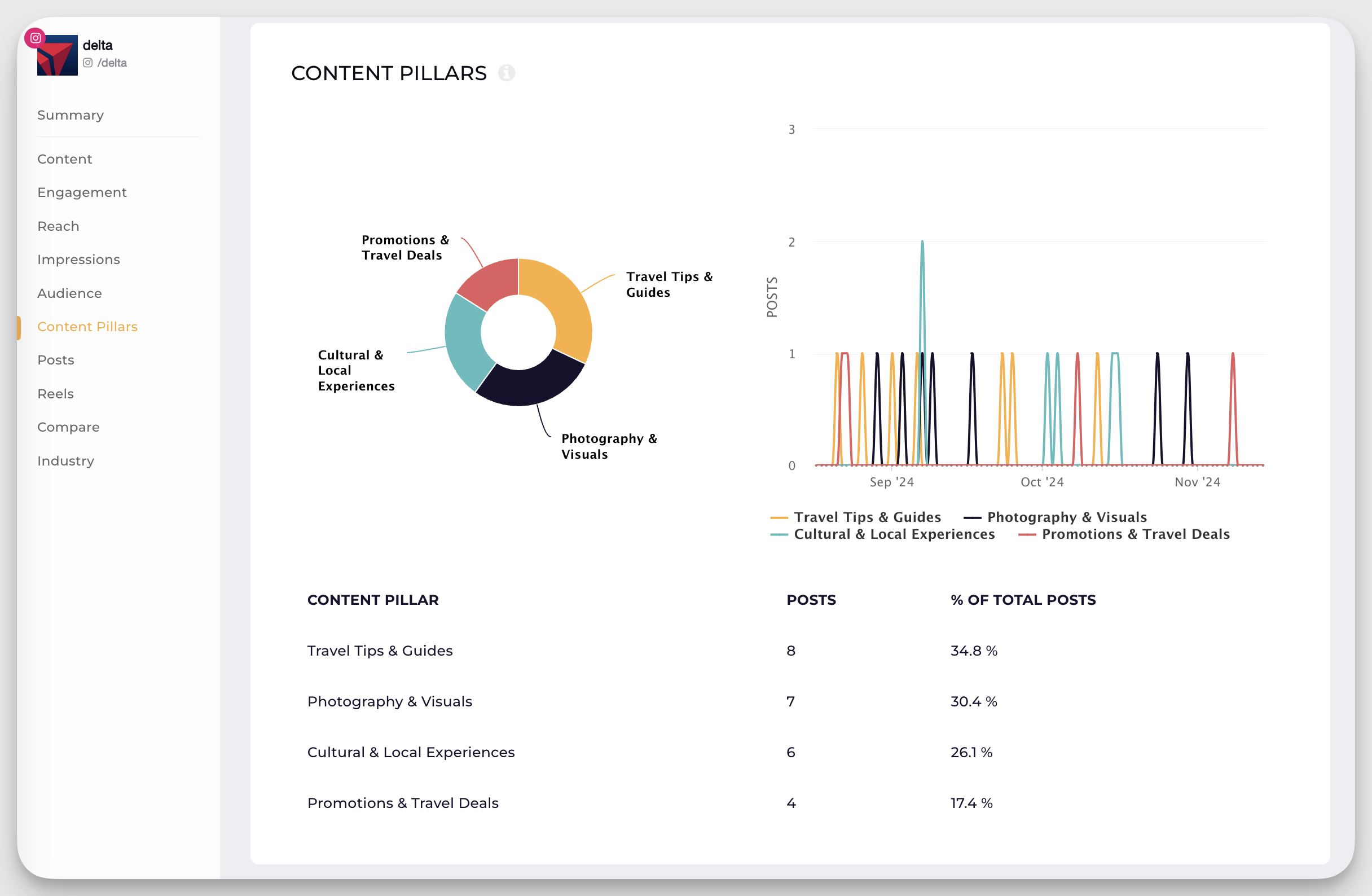
AnswerRocket (for business analytics)
AnswerRocket is a business analytics AI tool that transforms enterprise data analysis with its advanced machine learning and natural language processing capabilities. Its AI assistant, Max, allows users to easily interact with data through conversational AI, quickly uncovering actionable insights as if chatting with a colleague.
This approach greatly enhances analytics productivity, enabling teams to gain instant insights and stay ahead of business performance.
A key feature of AnswerRocket is its ability to automate recurring analyses, providing timely reports without manual effort.
Fully customizable to match your businessβs unique data needs, AnswerRocket integrates seamlessly with existing systems, empowering businesses to make faster, data-driven decisions with ease.
Coefficient (for data analytics)
Coefficient is an AI-powered data analytics tool that streamlines the management and analysis of live data within Google Sheets and Excel, allowing businesses to effectively use AI for data analytics.
By automatically importing data from sources like Salesforce and HubSpot, Coefficient eliminates the need for manual exports and outdated dashboards, providing businesses with real-time insights to drive informed decision-making.
A key feature of Coefficient is its ability to create live pivot tables and dashboards directly in spreadsheets, making data processing faster and more efficient.
With tools for data cleaning, auto-mapping fields, and seamless data export, Coefficient helps businesses optimize their data analytics workflow, reducing time spent on data management and ensuring accurate, up-to-date insights.
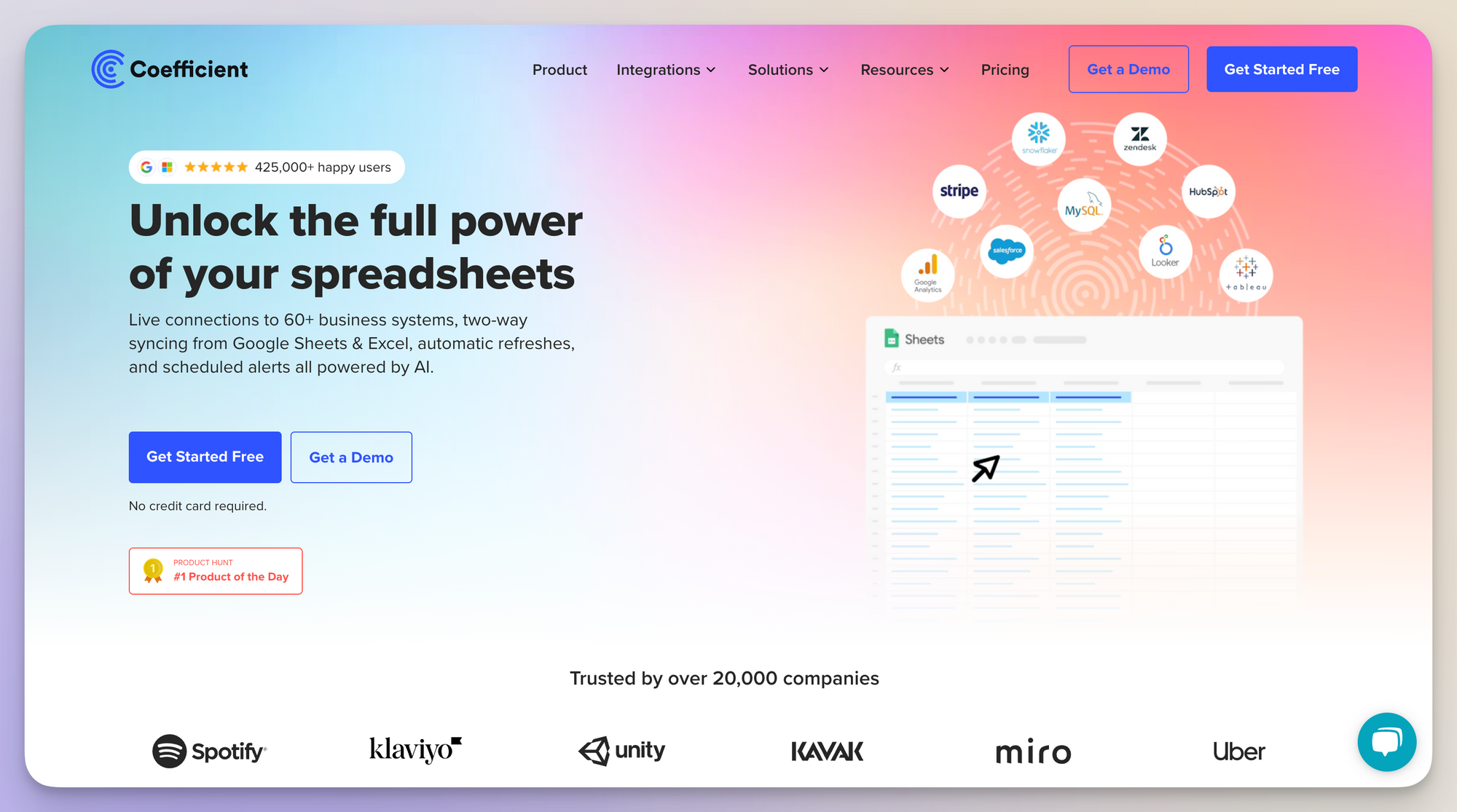
Power BI (for business analytics)
Power BI is a powerful AI business analytics tool from Microsoft that enables organizations to visualize and share insights from their data.
With its intuitive interface and robust integration capabilities, Power BI allows users to connect to various data sources, transform raw data into interactive dashboards, and generate detailed reports.
This makes it easier for businesses to monitor key metrics, identify trends, and make data-driven decisions quickly.
One of Power BI's strengths is its ability to handle large datasets and perform real-time analysis.
AI analytics helps alleviate a companyβs growth pains by efficiently handling large datasets from disparate sources. Whether you're pulling data from Excel, SQL databases, or cloud services, Power BI consolidates this information into a single, unified view, allowing for seamless data exploration
Additionally, Power BI's strong integration with other Microsoft tools, like Excel and Azure, enhances its utility across different business functions, making it a versatile choice for companies looking to harness the full potential of their data.
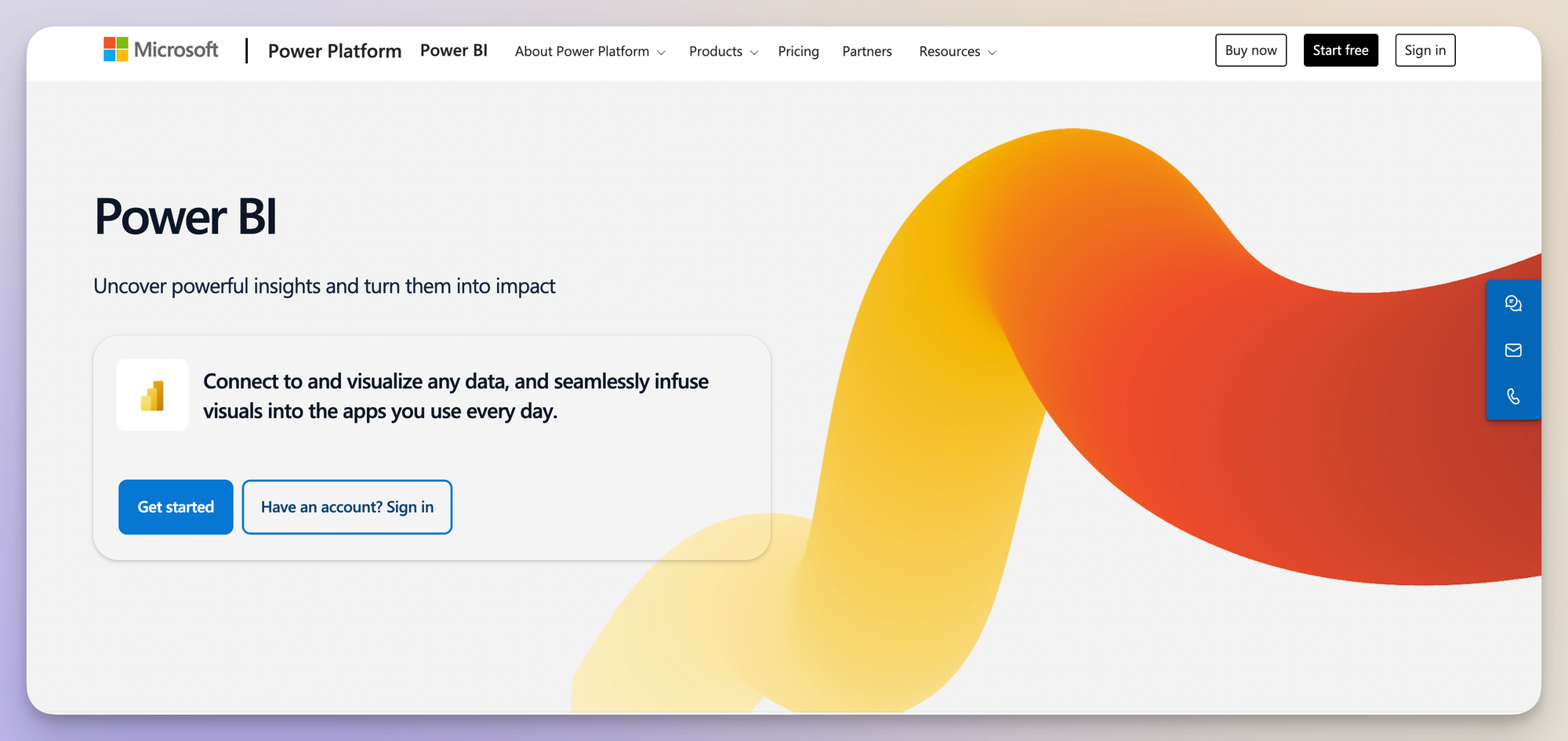
Tableau (for business visualization)
Tableau, a leading AI-powered analytics platform from Salesforce, transforms the way businesses visualize and interpret data.
Known for its intuitive interface and robust capabilities, Tableau allows users to connect to various data sources and create interactive, insightful visualizations that drive informed decision-making, especially when working with big data using advanced machine learning (ML).
With built-in AI and machine learning features, Tableau makes it easier than ever to explore data, identify trends, and share findings across teams.
One of Tableau's key strengths is its flexibility in deployment, whether in the cloud, on-premises, or integrated with Salesforce CRM. This versatility ensures that organizations can tailor Tableau to fit their specific needs, enhancing their data analysis processes.
Additionally, Tableau's emphasis on visual storytelling and collaboration helps teams across an organization stay aligned and make data-driven decisions with confidence, making it a preferred choice for businesses aiming to foster a strong data culture.
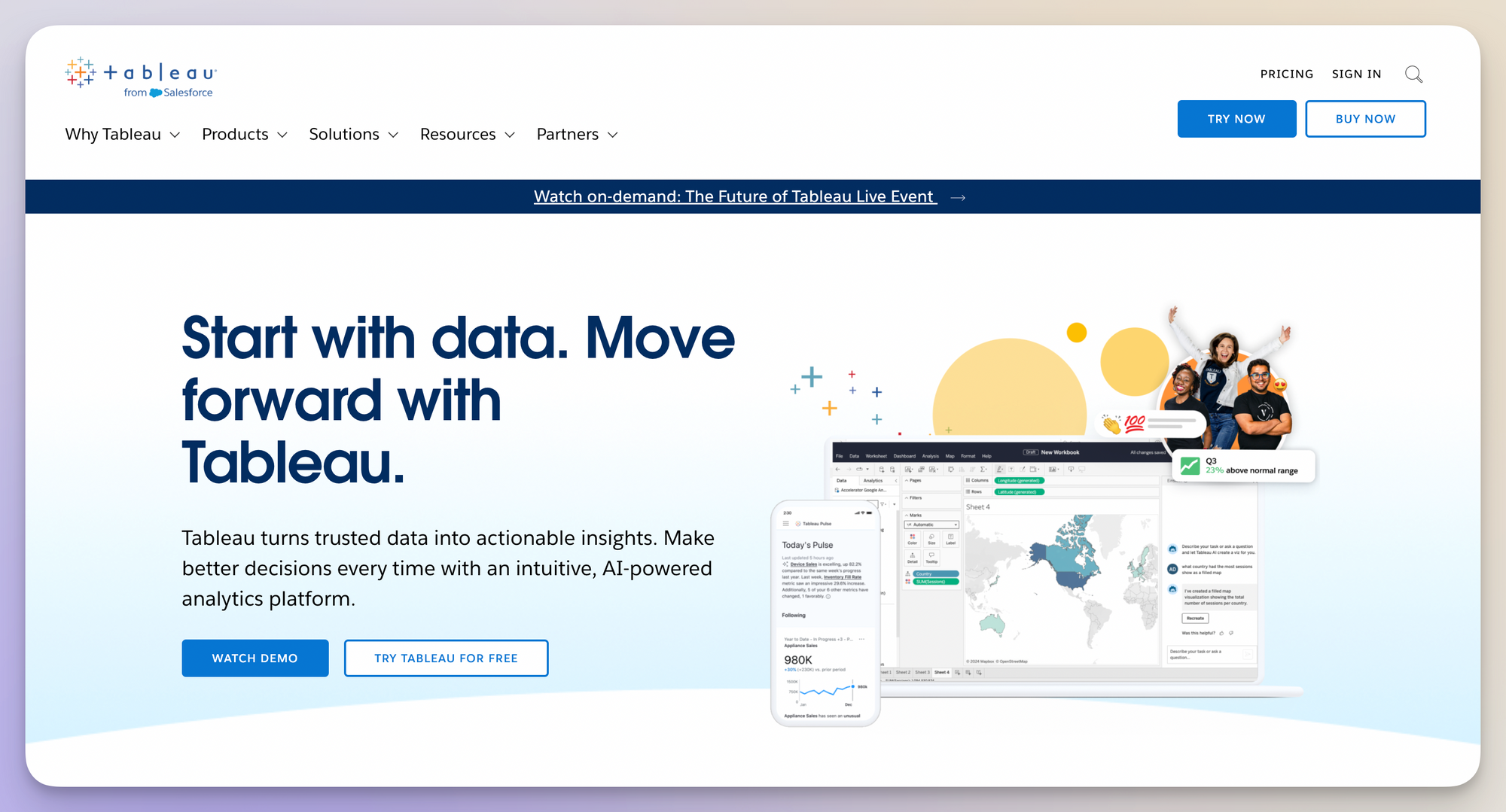
Polymer (for customizable dashboards)
Polymer is an intuitive AI-powered analytics tool that transforms raw data into customizable dashboards and presentations with ease. Results from AI data analysis are seamlessly integrated, allowing users to connect data sources and automatically generate dashboards without requiring any technical expertise.
Whether you're working with data from Google Ads, Facebook Ads, or Google Analytics, Polymer streamlines the process of creating visually appealing and insightful reports, saving hours of manual effort.
A standout feature of Polymer is its conversational AI, PolyAI, which lets users ask questions in plain English and receive clear answers in seconds. This functionality makes data analysis accessible to non-experts, allowing anyone to explore data insights without the need for extensive training.
Additionally, Polymer offers seamless sharing and collaboration options, enabling teams to work together on data projects efficiently.
With its easy-to-use interface and powerful AI capabilities, Polymer is an ideal solution for businesses looking to simplify and enhance their data analytics processes.
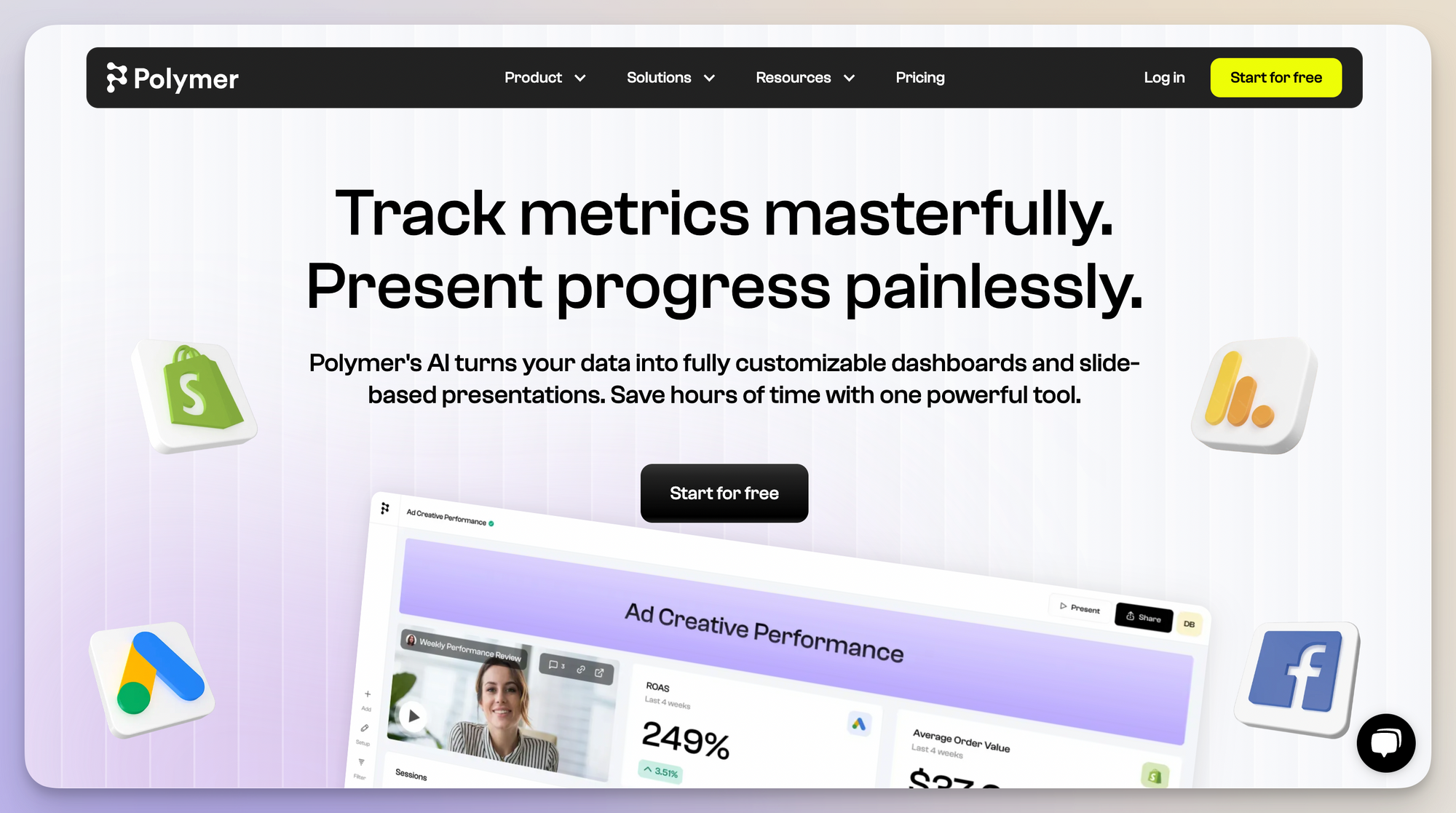
The future of AI analytics
The future of AI analytics is set to transform the marketing industry on a macro level, offering businesses unprecedented insights and the ability to optimize strategies with greater precision.
As AI-powered analytics tools continue to advance, they are becoming essential for marketing teams looking to stay competitive in an increasingly data-driven environment.
Personalization at scale
One of the most significant impacts of AI analytics in marketing is the ability to personalize campaigns at scale.
By analyzing vast amounts of customer data, including browsing behavior, purchase history, and social media interactions, AI can help marketers create highly targeted content that resonates with individual consumers.
This level of personalization, driven by AI data insights, enhances customer engagement and drives higher conversion rates.
Predictive analytics for campaign optimization
Data analytics and artificial intelligence are also transforming how marketers plan and execute campaigns.
With predictive and prescriptive analytics, businesses can forecast the success of marketing strategies by analyzing historical data and market trends.
For example, AI can predict which types of content will perform best, which channels are most effective, and even the optimal timing for campaign launches. This allows marketers to allocate resources more efficiently and maximize ROI.
Real-time marketing decisions
The ability to make real-time decisions is another area where AI analytics is making a significant impact. By continuously monitoring campaign performance, AI-powered analytics tools can provide instant insights into whatβs working and whatβs not.
For instance, if an ad campaign is underperforming, AI can quickly identify the issueβwhether it's the targeting, the creative, or the timingβand suggest adjustments on the fly. This agility ensures that marketing efforts remain effective and relevant, even as market conditions change.
As AI continues to evolve, its integration into marketing strategies will only deepen, allowing businesses to harness the full potential of AI analytics.
By using AI analytics to derive a stronger return on investment (ROI), companies can enhance customer experiences and optimize campaigns in real-time.
The future of AI in marketing, with diverse AI use cases in marketing, promises to drive more precise, impactful, and data-driven strategies that will give businesses a significant edge in a competitive landscape.
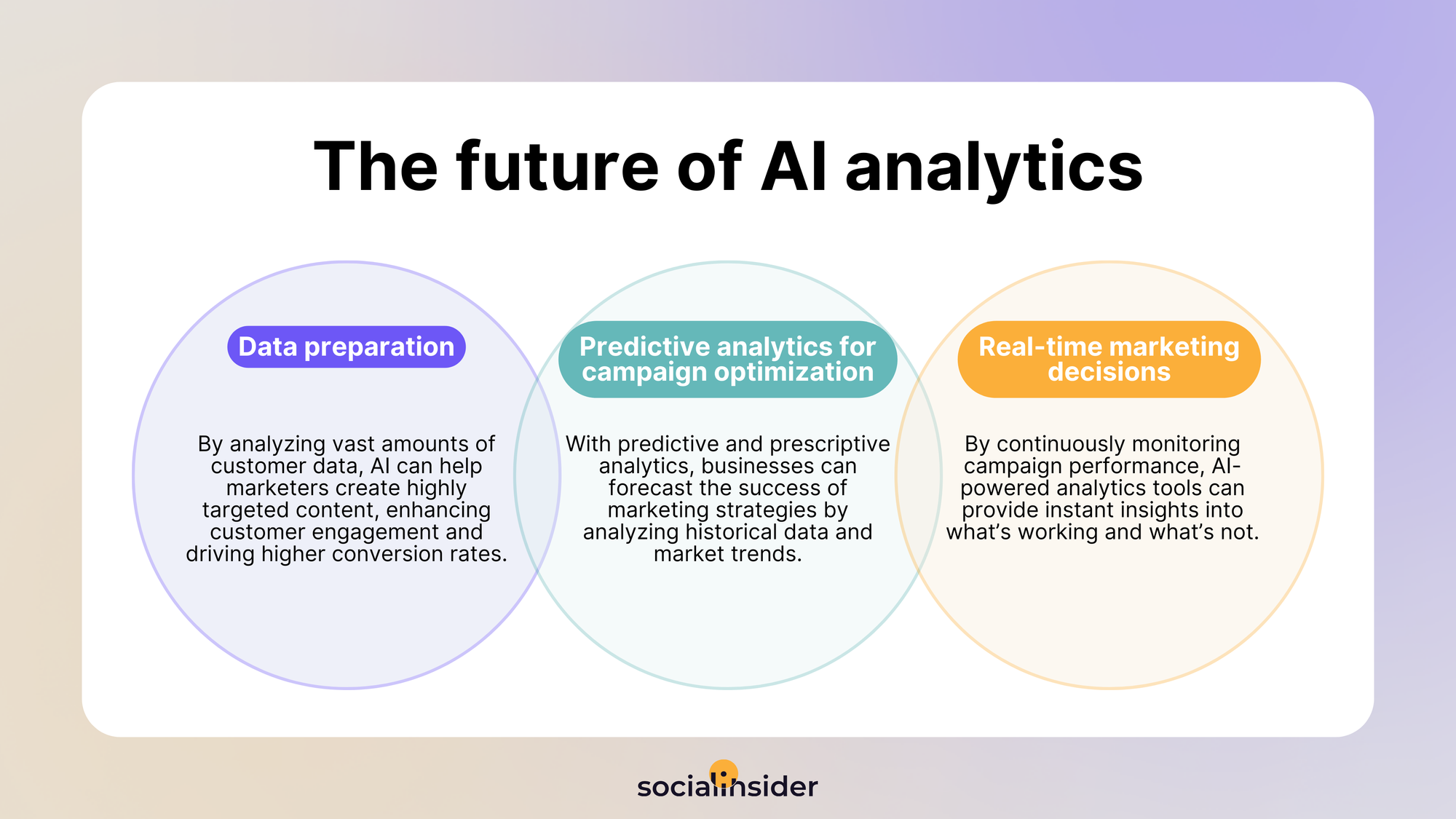
Final thoughts
AI analytics is rapidly becoming a cornerstone of modern business strategy, offering unparalleled insights and efficiency across various industries.
As we've explored, tools like Socialinsider are leading the charge in transforming how businesses approach data analysis, particularly in the realm of marketing.
Some common predictive analytics examples across different industries include forecasting customer churn in telecommunications, predicting equipment failures in manufacturing, and estimating future sales trends in retail.
By leveraging AI-powered analytics, companies can gain a deeper understanding of customer behavior, optimize their campaigns in real-time, and stay ahead in an increasingly competitive market.
The future of AI analytics promises even greater advancements, enabling businesses to scale personalization, predict campaign success, and make agile decisions that drive growth and innovation.
As AI continues to integrate more deeply into business operations, tools like Socialinsider will be indispensable for companies looking to maintain a competitive edge and achieve long-term success in a data-driven world.